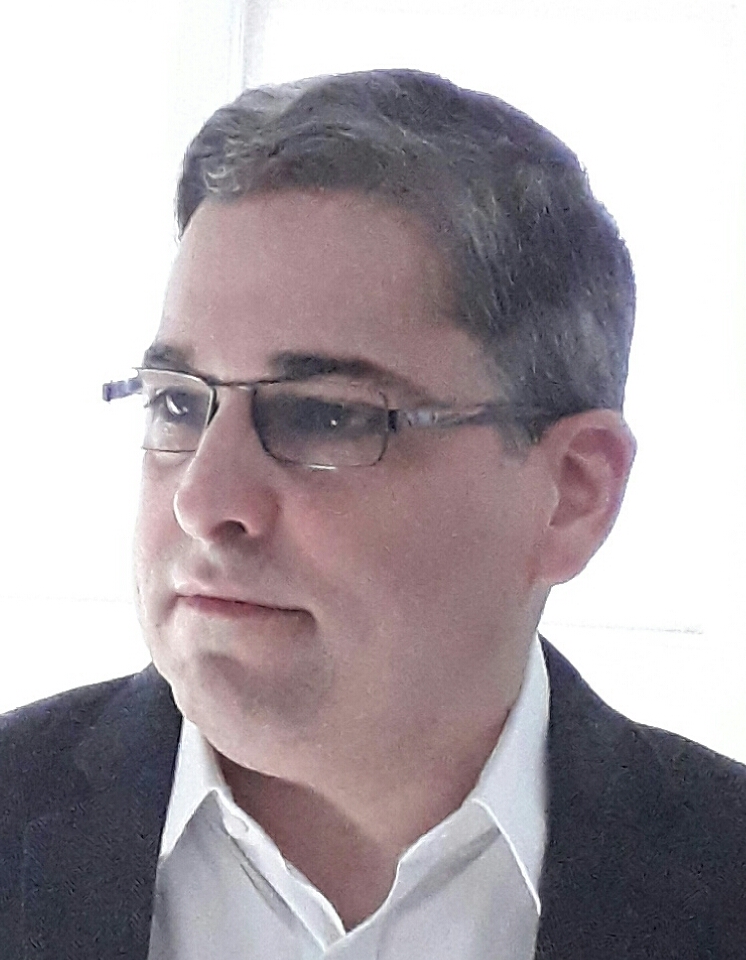
- Neural-Symbolic Computation, Neural Networks and Applied Logic, Complex Networks;
- Machine Learning, Integrating Robust Learning and Reasoning under Uncertainty;
- Cognitive Agents and Intelligent Systems, Knowledge Extraction, Visual Information Processing;
- Business Process Evolution, Requirements Engineering, Automated Software Engineering.
Invited Talks and Seminars:
Argumentation Neural Networks. Invited talk at Mallow'09 Argumentation Day, Educatorio della Provvidenza, Turin, Italy, September 2009.
Neurons and Symbols: A Manifesto, Dagstuhl Seminar on Learning Paradigms in Dynamic Environments. Wadern, Germany, July 2010.
Robust Logic Learning in Neural Networks, Invited talk at the Lab for Adaptive Systems, University of Luxembourg, July 2010.
Neural-Symbolic Computation, ICCL Summer School on Cognitive Science, Logic and Connectionism, TU Dresden, Germany, August 2010.
Neural-Symbolic Systems for Cognitive Reasoning, Invited talk at the School of Computing, University of Kent, UK, June 2011.
Neural-Symbolic Computation, The Future of Machine Learning Panel, Imperial College London, UK, September 2011.
Neural-Symbolic Systems for Cognitive Reasoning, Invited talk at the Informatics Colloquium, King's College London, October 2011.
Neural-Symbolic Systems for Cognitive Reasoning, Invited talk at the School of Computing, University of Leeds, UK, October 2011.
Neural-Symbolic Systems for Verification and Adaptation, Invited talk at the Dept. of Computing, Imperial College London, UK, March 2012.
Fast Relational Learning using Neural-Symbolic Systems, Invited talk at the Dept. of Computing, Imperial College London, UK, November 2013.
Neural-Symbolic Computing, Deep Logic Networks and Applications. Schloss Dagstuhl Seminar 14381, Wadern, Germany, September 2014.
Neural-Symbolic Learning and Reasoning: Contributions and Challenges. AAAI Spring Symposium, Stanford University, Palo Alto, March 2015.
Relational Knowledge Extraction from Neural Networks. Talk at NIPS Cognitive Computing workshop, Montreal, Canada, December 2015.
Neural-Symbolic Systems for Verification, Run-time Monitoring and Learning. Dept. of Computer Science, University of Oxford, UK, March 2017.
Neural-Symbolic Systems for Human-Like Computing. Dagstuhl Seminar on Human-Like Computing, Schloss Dagstuhl, Wadern, Germany, May 2017.
Avoiding Deep Horses: Finding Structure in Deep Networks. Invited talk at Horse 2017, Queen Mary University of London, September 2017.
Neurosymbolic Computation: Thinking Beyond Deep Learning. Invited talk, Department of Computing, Imperial College London, November 2017.
On the Need for Knowledge Extraction from Deep Networks. Invited talk, Data Science Institute, Imperial College London, February 2018.
Thinking Beyond Deep Learning? Neurosymbolic Computing. Invited talk, Cognitive Computation Symposium, City, University of London, February 2018.
Neural-Symbolic Systems for Verification, Run-Time Monitoring and Learning. Keynote at the 24th International Workshop on Algebraic Development Techniques, Royal Holloway University of London, UK, 2-5 July 2018.
Neurosymbolic Computing: Thinking beyond Deep Learning. Invited talk at IBM Research, Rio de Janeiro, Brazil, 12 July 2018.
Neural-Symbolic Learning I, ACAI Summer School on Statistical Relational Artificial Intelligence, Ferrara, Italy, September 2018.
Neural-Symbolic Learning II, ACAI Summer School on Statistical Relational Artificial Intelligence, Ferrara, Italy, September 2018.
Deep Learning and Symbolic Reasoning. Invited talk at the DeepMind CSML Seminar Series, UCL, London, November 2018.
Deep Learning and Symbolic Reasoning. Invited talk at the Department of Bioengineering, Imperial College London, November 2018.
Verification and Explainability of Neural Networks. Discussion Panel, Verification of Neural Networks, AAAI 2019 Spring Symposium, Stanford University, California, USA, 25 March 2019.
Neural-Symbolic Systems for Software Model Evolution, Run-Time Monitoring and Property Learning. Human-Like Computing Third Wave of AI Workshop (3AI-HLC 2019), Imperial College London, UK, April 2019.
Logic Tensor Networks. Keynote at ACDL Satellite Workshop on Graph Neural Networks, Advanced Course on Data Science and Machine Learning, Siena, Italy, 20 July 2019.
Continual Learning, Reasoning and Explainable AI through Knowledge Extraction from Neural Networks. Keynote at the Workshop on AI and ML in Finance, The Royal Society, London, Organised by the University of Cambridge, Judge Business School, 8 October 2019.
Logic Tensor Networks. Machine Learning Meetup, London, 23 October 2019.
Continual Learning, Reasoning and Explainable AI through Knowledge Extraction from Neural Networks. Keynote at Workshop on Explainability in Finance, The Alan Turing Institute, London, 31 October 2019.
Continual Learning and Explainable AI in Finance, Invited talk at AI UK, The Alan Turing Institute, London, UK, 24 March 2020.
Continual Learning and Explainable AI in Finance, Keynote talk at the Warwick Business School, Gillmore Centre for Financial Technology, Investment AI and Machine Learning Symposium, University of Warwick, Coventry, UK, 3 April 2020.
Neural-Symbolic AI. Invited talk at Samsung AI, Samsung Research, Cambridge, UK, May 2020.
Neural-Symbolic AI, Invited talk at King's College London, Department of Informatics, London, June 2020.
Neurosymbolic AI, Keynote talk at The Sixth International Conference on Machine Learning, Optimization and Data Science (LOD2020), Workshop on Integrative Machine Learning, Siena, Italy, July 2020.
Neurosymbolic AI, Seminar at Fundacao Getulio Vargas, Instituto de Matematica Aplicada (FGV-IMAp), Rio de Janeiro, Brazil, November 2020.
NeSy and Explainable AI. Tutorial at 15th International Workshop on Learning and Reasoning (NeSy'2021), Virtual, October 2021.
Trust in XAI Requires High Fidelity. Keynote Talk at XAI in Investment Management, Warwick Business School, University of Warwick, 19 November 2021.
From Knowledge Extraction to Accountability in AI. Invited talk at Fujitsu Research, Seminar Series on AI Ethics and Applications, Virtual, 25 November 2021.
From Knowledge Extraction to Accountability in AI, Invited Talk, School of Computing, University of Kent, 7 February 2022.
Neurosymbolic AI, Invited Talk, Department of Computer Science and Artificial Intelligence, University of Granada, Spain, 11 May 2022.
Neurosymbolic AI: The Third Wave. Keynote talk, Machine Learning and Logical Reasoning: The New Frontier, Schloss Dagstuhl Seminar 22291, Wadern, Germany, 18 June 2022.
Neurosymbolic AI. Keynote talk, IBM Research Summer School on Neuro-Symbolic AI, T.J. Watson Research Center, Yorktown Heights, USA, 8 August 2022.
Neurosymbolic Argumentation, Panel Discussion, Argumentation and Machine Learning Workshop at COMMA 2022, Cardiff University, UK, 13 September 2022.
Knowledge Extraction and Manipulation, 3rd Meeting of Researchers in Law and Sustainability, Universidade de Santa Cruz do Sul (UNISC), Rio Grande do Sul, Brazil, 21 September 2022.
Neurosymbolic AI, invited talk at the Digital Environment Research Institute, Queen Mary, University of London, 10 Nov 2022.
On the Need for Measurable Explainable AI in Finance, keynote talk at The Fintech Conference, City, University of London, 16 Dec 2022.
Sustainable AI, AI Debate 3, hosted by Gary Marcus and Vincent Boucher, Montreal.AI. Invited panellist alongside leading linguist Noam Chomsky, information economics pioneer Erik Brynjolfsson, IBM global ethics leader Francesca Rossi, Deep Learning pioneer Juergen Schmidhuber, leading AI entrepreneur Kai-Fu Lee, and best-selling AI author Gary Marcus, among others. 23 Dec 2022.
Reasoning in Neurosymbolic AI, IBM Watson Neuro-Symbolic AI Workshop 2023 (virtual), Jan 23 - 27, 2023.
On the Risk, Existential Risk and What is Left to Do in AI, Keynote talk and Discussion Panel, Alan Turing Institute 2nd Symposium of the Interest Group on Knowledge Graphs, City University of London, UK, 16 June 2023.
Neurosymbolic AI Contributions to Metalearning. Keynote talk at ECML/PKDD Workshop on Neurosymbolic Metalearning and AutoML, Turin, Italy, 18-22 Sep 2023.
IA Sustentavel. Invited talk at Instituto de Inteligencia Computacional Aplicada, UFES, Espirito Santo, Brazil, 17 Nov 2023.
Neurosymbolic AI to achieve Trustworthy AI. Keynote talk at Brazilian Symposium on Formal Methods, SBFM-2023, Manaus, Brazil, 4-8 Dec 2023.
Sustainable AI. Invited talk at NeurIPS 2023, Thirty-seventh Conference on Neural Information Processing Systems, ROAD-R 2023 challenge: the Road Event Detection with Requirements Challenge, New Orleans, LA, 15 Dec 2023.
What is neural-symbolic computation?
Humans are constantly learning and reasoning in order to make decisions as part of a permanent cycle of knowledge acquisition. In this process, learning and reasoning are almost indistinguishable. However, they are typically studied separately in Artificial Intelligence, leading to computational systems where the emphasis is on either aspect of cognition, but not on the interplay between them.
Our research work brings the modelling of learning and reasoning together with the use of neural-symbolic integration. Neural-symbolic systems can produce better models of knowledge acquisition, robust learning and reasoning under uncertainty. In the longer term, it is expected that they will offer a better understanding of such fundamental phenomena of cognition.
Neural-symbolic systems integrate logical reasoning and statistical learning by offering sound translation algorithms between network and logic models. They contain three main components: (1) knowledge representation and reasoning in neural networks, (2) knowledge evolution and network learning, and (3) knowledge extraction from trained networks.
In a neural-symbolic system, neural networks provide the machinery for efficient computation and robust learning, while logic provides high-level representations, reasoning and explanation capabilities to the network models, promoting modularity, facilitating validation and maintenance and enabling a better interaction with existing systems.
Neural-symbolic systems have important applications in diverse areas such as bioinformatics, fraud prevention, assessment and training in simulators, cognitive robotics, general game playing, image, audio and video classification, software verification and the semantic web.
In a nutshell, neural-symbolic systems seek to benefit from the knowledge representation and reasoning capacities of applied logic, and the learning capacities of neural networks, enabling effective learning from noisy data and online reasoning about what has been learned.